Columbia University
Irving Medical Center
Neurological Institute
710 West 168th Street, 3rd floor
(212) 305-1818


TaubCONNECT Research Perspective:
January 2024
2: ZCCHC17 Modulates Neuronal RNA Splicing and Supports Cognitive Resilience in Alzheimer's Disease
3: Benchmarking of Deep Neural Networks for Predicting Personal Gene Expression from DNA Sequence Highlights Shortcomings
![]() | ![]() | |
Yian Gu, MD, MS, PhD | Lawrence S. Honig, MD, PhD |
Blood-based biomarkers, such as amyloid beta (Aβ), tau, neurofilament light chain (NfL), and glial fibrillary acidic protein (GFAP), provide insights into the molecular signatures of Alzheimer’s disease (AD) and related dementias (ADRD). These biomarkers offer advantages over cerebrospinal fluid and positron emission tomography biomarkers due to their non-invasive nature, ease of access, affordability, and suitability for large-scale epidemiological studies and clinical trials. The clinical utility of these biomarkers extends to diagnosis, disease tracking, prognosis, treatment management, screening, early detection, and risk assessment.
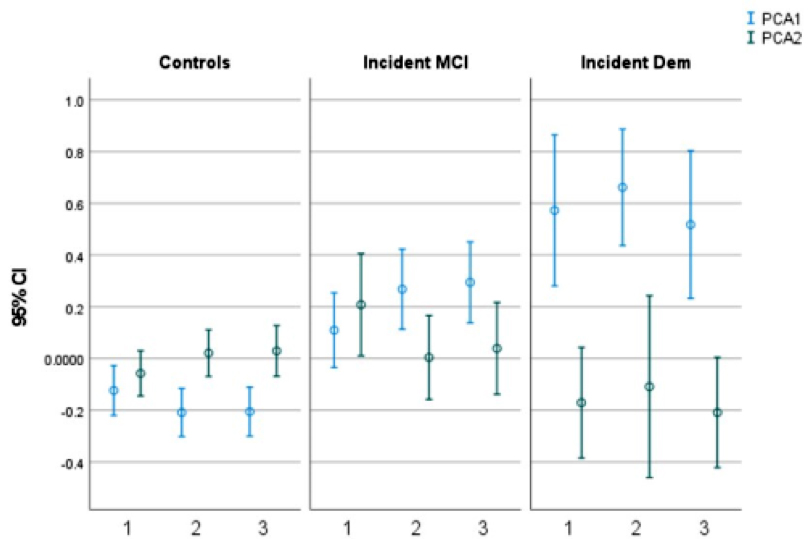
Figure 2. Two patterns (PCA1 and PCA2) derived from principal component analyses of the blood-based biomarkers at the three clinical visits over the period of follow-up. Mean (95% confidence interval [CI]) of the biomarker pattern scores (Y axis) at visits 1, 2, and 3 (X axis), in cognitively unimpaired, incident mild cognitive impairment (MCI), and incident dementia (Dem) participants. Blue represents PCA1, and green represents PCA2.
Research on these biomarkers has primarily been centered on their diagnostic capability in both research and specialized clinical settings. Data from select participants have informed the development of threshold levels for distinguishing the presence or absence of AD pathology, using autopsy findings or amyloid PET imaging as reference standards. Despite this, universally accepted diagnostic thresholds for these biomarkers have not been established, nor have they been broadly applied in monitoring disease progression or evaluating treatment responses. Moreover, these biomarkers show promise in identifying cognitively unimpaired individuals at risk of dementia, and in predicting mild cognitive impairment (MCI), AD, or ADRD in asymptomatic individuals. Tracking longitudinal changes in biomarker levels in diverse, large-scale population groups could enhance the value of these biomarkers.
Using data from the multiethnic Washington Heights–Hamilton Heights–Inwood Columbia Aging Project (WHICAP), we explored the potential of initial blood-based biomarker measurements to predict later diagnosis of MCI or AD. Additionally, we investigated whether changes in these biomarkers over time varied between cognitively unimpaired individuals and those with newly diagnosed MCI or AD. As recently reported in Alzheimer’s & Dementia, we found that higher initial p-tau181 to Aβ42 ratios were linked to a heightened risk of developing AD. Conversely, a biomarker profile marked by high Aβ42 to Aβ40 ratios but low p-tau181 to Aβ42 ratios was associated with a reduced risk of dementia. Compared to CU, participants who developed MCI or dementia had a faster decrease in this protective biomarker pattern, reflecting AD-specific pathological change. Larger studies or meta-analyses are needed to examine whether the predictive utility of blood-based biomarkers for AD differs across racial/ethnic groups.
Yian Gu, MD, MS, PhD
Associate Professor of Neurological Sciences (in Neurology, Epidemiology, the Gertrude H. Sergievsky Center, and the Taub Institute) at CUMC
yg2121@cumc.columbia.edu
Lawrence S. Honig, MD, PhD
Professor of Neurology (in The Taub Institute, The Sergievsky Center)
lh456@cumc.columbia.edu

ZCCHC17 Modulates Neuronal RNA Splicing and Supports Cognitive Resilience in Alzheimer's Disease
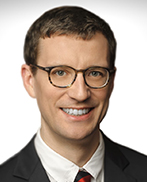
Andrew F. Teich, MD, PhD
RNA splicing has been shown by several laboratories to be abnormal in Alzheimer’s disease, but the mechanism for this is not well understood. In Bartosch et al., our team from the Taub Institute, including Drs. Lee, Klein, Vardarajan, De Jager, and Sproul, show that ZCCHC17, a previously identified master regulator of synaptic dysfunction in AD, plays a role in neuronal RNA splicing. As recently reported in The Journal of Neuroscience, motivated by these data, we then show that ZCCHC17 dysfunction is sufficient to explain some of the RNA splicing abnormalities seen in AD brain tissue, including synaptic gene splicing abnormalities. Using data from human patients, we also demonstrate that ZCCHC17 mRNA levels correlate with cognitive resilience in the setting of AD pathology. The role of ZCCHC17 in splicing is further supported by showing that ZCCHC17 interacts with an array of RNA binding proteins, including splicing proteins (see Figure 1D). Interestingly, approximately half of these same proteins interact with tau, and our study further shows similarities between splicing abnormalities in ZCCHC17 knock-down neurons and tau overexpressing neurons.
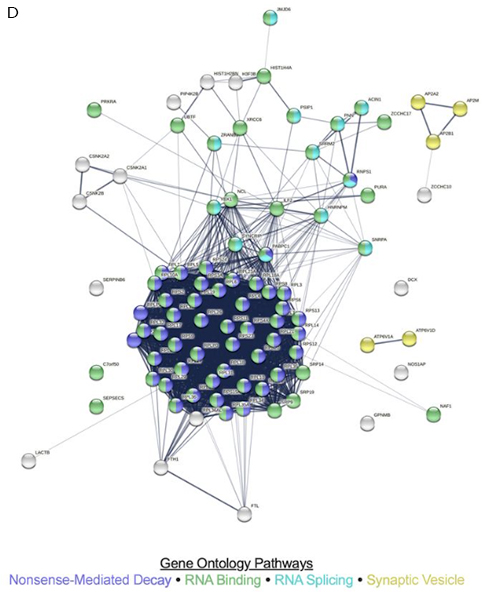
Figure 1D. STRING-derived known and predicted protein–protein interactions among ZCCHC17 binding partners, with relevant strongly enriched gene ontology pathways highlighted. ZCCHC17 binding partners were defined as the union of significant hits from both LC-MS/MS experiments and comprise 91 unique proteins in total.
These results suggest that maintenance of ZCCHC17 function may be a therapeutic strategy for supporting cognitive function in AD patients, possibly through maintenance of normal RNA splicing of synaptic genes. They also demonstrate that tau may be a mediator of ZCCHC17 dysfunction, possibly in part due to sequestration of ZCCHC17 interactors in an environment of high intracellular tau. More generally, this study motivates future work examining the role of abnormal RNA processing in AD-related cognitive decline.
Andrew F. Teich, MD, PhD
Associate Professor of Pathology and Cell Biology (in Neurology)
aft25@cumc.columbia.edu

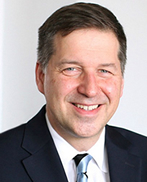
Philip L. De Jager, MD, PhD
Recently, deep learning techniques have become leading-edge in various tasks related to regulatory genomics, including gene expression prediction from genomic DNA. These methods are increasingly recognized as vital tools for interpreting the wide range of genetic variations found in individual genomes. Although previous evaluations have focused on how well these methods predict gene expression across different genomic areas, there's a noticeable gap in systematic benchmarking for their effectiveness in predicting across different individuals, which is crucial for their role in personal genome interpretation.
Recently, as part of a collaborative investigative team led by academic colleagues Drs. Maria Chikina (University of Pittsburgh) and Sara Mostafavi (University of Washington), we utilized whole genome sequencing and gene expression data from 839 individuals in the ROSMAP study to assess how current methods perform in predicting gene expression variations among individuals at different genetic sites. As recently reported in Nature Communications, our findings indicate that existing sequence-based deep learning models, which are trained using a single reference genome as input-output pairs, often inaccurately predict how single nucleotide variants (SNVs) affect gene expression. Additionally, we discovered that these neural network models are outperformed by simpler methods, like PrediXcan, when it comes to predicting the effects of genetic variations among individuals.
Looking ahead, we propose that new models should be evaluated not only based on genome-wide metrics of the absolute sizes of causal eQTL effects but also on their accuracy per gene in matching both the direction and magnitude of the effects of causal variants as predicted and observed.
Philip L. De Jager, MD, PhD
Weil-Granat Professor of Neurology (in the Taub Institute)
pld2115@cumc.columbia.edu
